A new financial research paper has been published and is related to cryptocurrency trading strategies:
Author: Jo, Park, Shefrin
Title: Bitcoin and Sentiment
Link: https://papers.ssrn.com/sol3/papers.cfm?abstract_id=3230572
Abstract:
On the surface, cryptocurrencies share important features in common with high sentiment beta stocks. Baker and Wurgler (2007) identify high sentiment betas with small startup firms that have great growth potential. This paper investigates the degree to which, during the period July 18, 2010 to February 26, 2018, the return to bitcoin displayed the characteristics of a high sentiment beta stock. Using a sentiment-dependent factor model, the analysis indicates that in large measure, bitcoin returns resembled returns to high sentiment beta stocks.
Notable quotations from the academic research paper:
"The main objective of this paper is to investigate the degree to which bitcoin resembles a “high sentiment beta” stock, a term introduced by Baker and Wurgler (2007). They note that some stocks are more vulnerable to being mispriced than others, stating: “stocks of low capitalization, younger, unprofitable, high-volatility, non–dividend paying, growth companies … are likely to be disproportionately sensitive to broad waves of investor sentiment.” This statement implies that when investors become excessively optimistic about stocks in general, they become even more optimistic about stocks of small firms that, while not currently profitable, are perceived as holding great potential for future profitability. Baker and Wurgler describe stocks that are disproportionately sensitive to investor sentiment as featuring “high sentiment beta.”
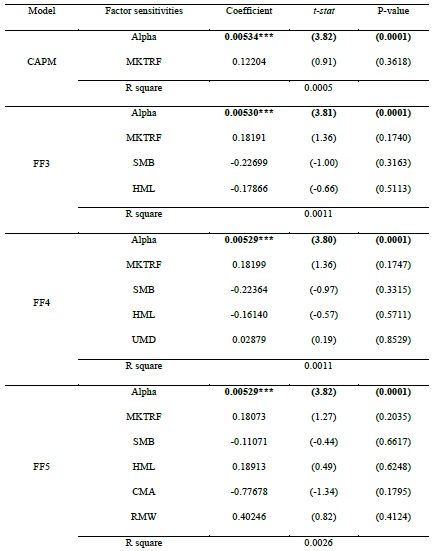
Table 3 presents the coefficient estimates of CAPM, Fama-French three, Carhart four, and Fama-French five factor models for U.S. daily excess returns on excess Bitcoin Return. We note that Jensen’s alpha is significant and positive at the 0.1% significance level in all the above asset pricing models during our sample period. Notice too that all of the coefficients of CAPM and Fama-French market and other factors are insignificant, suggesting that bitcoin returns are largely nonsystematic, at least from the perspective of a traditional factor pricing model.
There are at least three different channels by which sentiment can impact bitcoin returns. The first channel is bitcoin-specific, which is reflected in bitcoin’s Jensen’s alpha. The second channel involves the sensitivity of bitcoin’s price to general market sentiment. Baker and Wurgler (2007) describe such sentiment as general optimism about stocks.
The third channel involves the manner in which sentiment mediates fundamental factor loadings, as noted by Baker and Wurgler (2006). Of special interest is the impact of sentiment on factor loadings associated with size (SMB) and profitability (RMW), because of the analogy between bitcoin and Baker and Wurgler’s association of high sentiment beta to small startup firms that are not yet profitable but possess great growth potential. Because bitcoin is the cryptocurrency most closely associated with a blockchain technology, and blockchain activity is positively related to general economic activity, our prior expectation is that bitcoin returns will be statistically related to the market risk premium.
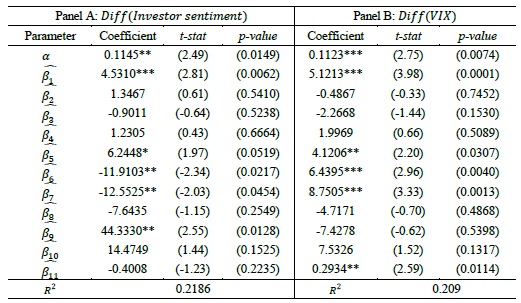
The results displayed in Table 5 Panel A indicate the following. First, the returns to bitcoin are statistically related to the market risk premium. Moreover, the interaction term involving the market risk premium features a negative coefficient. Therefore, when sentiment declines, bitcoin returns become more sensitive to the market risk premium.
Factor loading estimates for two other interaction terms are statistically significant. The first pertains to size (SMB), with a negative sign, and the second pertains to investment (CMA), with a positive sign. The bitcoin size effect is that when sentiment declines, bitcoin returns share a common feature with the stocks of small firms. This finding has the same flavor as Baker and Wurgler’s finding that the size effect only applies in connection with periods of negative sentiment. In particular, this finding is in line with the intuitive association of new cryptocurrencies to the stocks of small startups. The bitcoin investment effect is that when sentiment declines, bitcoin returns share a commonality with firms that invest aggressively rather than conservatively. In this respect, aggressive investment reduces firms’ free cash flows and equity returns, whose effect on bitcoin is most pronounced during times in which investors generally become more bearish.
Table 5 Panel A indicates that bitcoin returns are statistically related to the coefficient on the Fama-French profitability factor RMW ( 5 ), and with a positive sign. Therefore, the return to holding bitcoin is generally positive during periods when the stocks of higher profitability firms outperform the stocks of lower profitability firms. At first glance, this finding appears to be at odds with bitcoin returns resembling the returns to the stocks of unprofitable firms. However, in interpreting this finding, it is important to keep in mind that the return to small growth stocks cannot be explained by the Fama-French three-factor model, as those stocks have historically earned low returns, not the high returns predicted by the model. Similarly, in the extended Fama-French factor model, profitability is related differently to small, growth stocks than to other stocks.
One of the main implications of the regression analysis above is that sentiment impacts bitcoin returns indirectly through traditional factors, but with no direct discernable direct effect. This leaves open the question of how bitcoin returns and sentiment have coevolved over time. To investigate this issue, we employ Vector Auto Regression (VAR) models that focus on the dynamic relationship between Investor sentiment index and Bitcoin Return.
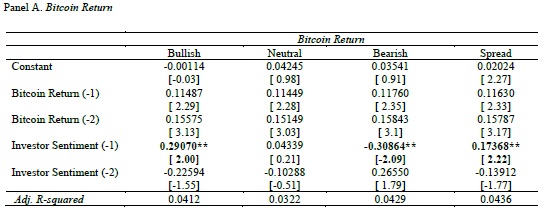
The results suggest that while Bitcoin Returns do not Granger-cause Investor Sentiment Index for the Investor Sentiment equations (Panel B), Investor Sentiment Index does Granger-cause Bitcoin Returns for the ratio of the Bitcoin Returns equation (Panel A). In particular, bullish (bearish) investor sentiment significantly drives Bitcoin Returns positively (negatively). It is noteworthy that although the time period of the bitcoin price decrease (i.e., January-February, 2018) is much shorter, and the percentage of bearish investor sentiment is somewhat lower, the negative impact of bearish investor sentiment on Bitcoin Returns seems to be a bit larger than the positive impact of bullish investor sentiment on Bitcoin Returns.
We further conduct VAR analysis with alternative measure of investor sentiment, Volatility Index (VIX) for robustness, and present the results in Table 9.

In Bitcoin Return equation, the coefficient on VIX (lagged 1) is significantly negative, which implies that a change in VIX index from day t–30 to day t negatively affects bitcoin return. This negative impact of VIX on Bitcoin Returns can be interpreted to mean that investors’ fear about the future market tends to induce a decrease in the price of bitcoin. Intuitively, when the volatility index increases, investors grow afraid of increased volatility, and sell bitcoin, lowering the bitcoin price. Expressed differently, if the VIX decreases, then the market is less volatile, and investors become willing to take risks to earn above average returns by buying bitcoin, thereby driving up its price."
Are you looking for more strategies to read about? Check http://quantpedia.com/Screener
Do you want to see performance of trading systems we described? Check http://quantpedia.com/Chart/Performance
Do you want to know more about us? Check http://quantpedia.com/Home/About
Follow us on:
Facebook: https://www.facebook.com/quantpedia/
Twitter: https://twitter.com/quantpedia