Once again, an interesting academic paper related to multiple equity factors:
Authors: Briere, Szafarz
Title: Factor Investing: The Rocky Road from Long Only to Long Short
Link: https://papers.ssrn.com/sol3/papers2.cfm?abstract_id=2908491
Abstract:
The performances of factor investing rely heavily on short sales, not only for building the initial long-short strategy, but also for regularly rebalancing the positions. Since short selling is subject to both legal restrictions and substantial costs, this paper examines how severely restrictions on short positions affect the financial attractiveness of factor investing. To fill the gap between unconstrained long-short allocations and restricted long-only portfolios, we consider two in-between strategies: the first imposes that only the market can be shorted, and the second is the so-called “130/30” scenario that caps total short exposure at 30%. The takeaways are twofold. First, any infringement to the long-short strategy can harm significantly the mean-variance performances of efficient factor-based portfolios. This is linked to the fact that the total short exposure of optimal long-short portfolios can reach figures around 400% and above. Second, the factor portfolios built originally by Fama and French (1992) with the purpose of developing asset pricing are impressively clear-sighted when it comes to portfolio management. Indeed, combining these portfolios generates mean-variance performances similar to those of optimized long-short portfolios, except for low levels of volatility.
Notable quotations from the academic research paper:
"A sizeable literature on portfolio management suggests that making short sales on a regular basis to rebalance portfolios is difficult. The aim of this paper is to assess the actual dependence of the mean-variance performances of factor investing on short selling restrictions.
In order to relax the necessity of short selling in factor investing, we proceed in two steps. First, we disentangle the long and short legs of the five historical factors. The ten resulting long-only factors provide additional flexibility in portfolio management. Second, short-selling restrictions, if any, are imposed separately on each of these ten factors. Last, we consider short-selling restrictions on the market index separately to reflect that shorting the market is much easier to implement (through derivative markets, for instance) than shorting any other factor.
Using as a benchmark the efficient frontier built from the Fama-French portfolios, we will examine the consequences on mean-variance performances of imposing five sets of short-selling-based restrictions.
Portfolios in group 1 (global long-only) exclude any short position whatsoever. Group 2 (longshort market + long-only factors) puts no restriction on exposure to market but excludes short positions in factors. The rationale is that easy access to index trading makes shorting the market easier and less costly than shorting factors, which are hardly tradable. Group 3 includes the typical 130/30 portfolios defined by the combination of a 130% long position and a 30% short one. Finally, in group 4, no position is constrained.
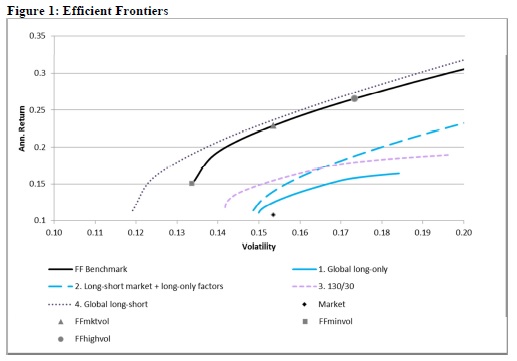
Figure 1 shows our five efficient frontiers. It reveals that the expected dominances are logically represented graphically. From definitions, we expect that the frontier corresponding to the global long-short case (group 4) dominates all the others, including the benchmark, since it allows running fully unconstrained optimization. Likewise, the global long-only case (group 1) is evidently more restrictive than both the cases of the long-short market + long-only factors (group 2) and 130/30 (group 3), which implies that the frontier associated with group 1 must be dominated by the two others. There is no clear dominance to be expected between the frontiers corresponding to groups 2 and 3, since on the one hand the exposure to market is unconstrained in group 2 but constrained by the 130/30 restriction in group 3, and the other factors can be shorted (to a certain extent) in group 3 but not at all in group 2. Hence, comparing the frontiers obtained for groups 2 and 3 can bring insights on the trade-off arising from shorting the market only versus shorting single-legged factors.
The market portfolio is located below all our frontiers of interest; even the most restricted one (corresponding to group 1), which bans any short sale."
Are you looking for more strategies to read about? Check http://quantpedia.com/Screener
Do you want to see performance of trading systems we described? Check http://quantpedia.com/Chart/Performance
Do you want to know more about us? Check http://quantpedia.com/Home/About